News
Deep learning model to identify new classes of antibiotic candidates
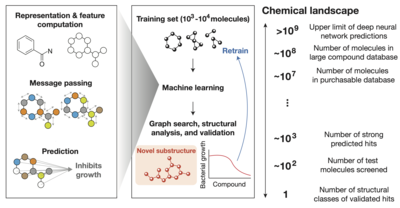
Bacterial resistance to existing antibiotics is a global threat to human health that will kill 10 million infected people per year by 2050, highlighting the need to discover new antibiotics. The conventional development of antibiotics is slow and has stalled with very few candidates reaching the market, increasing the need for new technologies to discover antimicrobial molecules. One promising avenue to explore and identify new antibiotics is the use of artificial intelligence.
In a joint effort, research teams from MIT, Harvard University and the Leibniz Institute of Polymer Research Dresden (IPF) used a type of artificial intelligence known as deep learning to find new structural classes of antibiotics. The approach is based on a black-box model that can learn to identify chemical structures associated with antimicrobial activity.Once an active structural feature is identified, the model explores millions of compounds and generates predictions for antimicrobial activity.
Wong et al. trained deep learning models with approximately 39,000 compounds for antimicrobial activity, with additional information on the chemical structure, and tested positive hits for functionality against bacteria and toxicity to human cells. Several compounds were discovered that killed methicillin-resistant Staphylococcus aureus in conventional growth assays and mouse models with low toxicity to human cells. This groundbreaking work is expected to not only identify compounds capable of killing pathogenic bacterial species, but also to predict novel antibiotic compounds to be synthesized de novo.
The IPF team was particularly involved in understanding the modes-of-action of the identified compounds using a range of single-cell techniques, specifically, single cell microscopy, atomic force microscopy and scanning electron microscopy.
“Discovery of a structural class of antibiotics with explainable deep learning”.
Nature – https://www.nature.com/articles/s41586-023-06887-8
Felix Wong1,2,3, Erica J. Zheng1,4,5, Jacqueline A. Valeri1,2,5, Nina M. Donghia5, Melis N. Anahtar1, Satotaka Omori1,3, Alicia Li3, Andres Cubillos-Ruiz1,2,5, Aarti Krishnan1,2, Wengong Jin6, Abigail L. Manson1, Jens Friedrichs7, Ralf Helbig7, Behnoush Hajian8, Dawid K. Fiejtek8, Florence F. Wagner8, Holly H. Soutter8, Ashlee M. Earl1, Jonathan M. Stokes1,2,9, Lars D. Renner7 & James J. Collins1,2,5
1) Infectious Disease and Microbiome Program, Broad Institute of MIT and Harvard, Cambridge, MA, USA.
2) Institute for Medical Engineering and Science and Department of Biological Engineering, Massachusetts Institute of Technology, Cambridge, MA, USA.
3) Integrated Biosciences, San Carlos, CA, USA.
4) Program in Chemical Biology, Harvard University, Cambridge, MA, USA.
5) Wyss Institute for Biologically Inspired Engineering, Harvard University, Boston, MA, USA.
6) Eric and Wendy Schmidt Center, Broad Institute of MIT and Harvard, Cambridge, MA, USA.
7) Leibniz Institute of Polymer Research and the Max Bergmann Center of Biomaterials, Dresden, Germany.
8) Center for the Development of Therapeutics, Broad Institute of MIT and Harvard,
9) Department of Biochemistry and Biomedical Sciences, Michael G. DeGroote Institute for Infectious Disease Research and David Braley Centre for Antibiotic Discovery, McMaster University, Hamilton, Ontario, Canada.
Deep-Learning-Modell zur Identifizierung neuer Klassen von Antibiotikakandidaten
Bakterielle Resistenz gegen etablierte Antibiotika stellt eine globale Bedrohung für die menschliche Gesundheit dar, die bis 2050 schätzungsweise 10 Millionen Todesfälle pro Jahr verursachen wird, was die Notwendigkeit der Entdeckung neuer Antibiotika unterstreicht. Da die konventionelle Entwicklung von Antibiotika nur langsam voranschreitet, werden innovative Technologien benötigt, um neue antimikrobielle Moleküle zu identifizieren: Der Einsatz künstlicher Intelligenz ist hier ein vielversprechender Lösungsansatz.
In einer gemeinsamen Arbeit von Forscherteams des MIT, der Harvard University und des Leibniz-Instituts für Polymerforschung Dresden (IPF) wurden datenwissenschaftliche Methoden des sogenannten "Deep Learning“ eingesetzt, um neue strukturelle Klassen von Antibiotika zu identifizieren. Der Ansatz basiert auf einem lernfähigen Black-Box-Modell zur Identifizierung chemischer Strukturen, die mit antimikrobieller Aktivität assoziiert sind. Wird ein aktives Strukturmerkmal identifiziert, untersucht das Modell Millionen von Verbindungen und erstellt Vorhersagen zu deren antimikrobiellen Aktivität.
Wong et al. trainierten ein Deep-Learning-Modell mit ca. 39.000 Verbindungen auf antimikrobielle Aktivität, wobei zusätzliche Informationen zur chemischen Struktur zur Verfügung gestellt wurden, und testete Treffer auf ihre Funktionalität gegen Bakterien und ihre Toxizität gegenüber menschlichen Zellen. Dabei wurden Moleküle identifiziert, die Methicillin-resistente Staphylococcus aureus (MRSA) in konventionellen Wachstumstests und Mausmodellen abtöten können und gleichzeitig eine geringe Toxizität gegenüber menschlichen Zellen aufweisen. Es wird erwartet, dass diese bahnbrechende Arbeit nicht nur zur Identifizierung weiterer Verbindungen führen wird, die in der Lage sind, andere pathogene Bakterienarten abzutöten, sondern auch zur Vorhersage von neuartigen antibiotischen Verbindungen, welche de novo synthetisiert werden können.
Das IPF-Team konzentrierte sich insbesondere auf das Verständnis der Wirkungsweise der identifizierten Moleküle auf Einzelzellebene unter Verwendung von Einzelzellmikroskopie, Rasterkraftmikroskopie und Rasterelektronenmikroskopie.
11.01.2024