Multivariate data analysis (MVDA) refers to methods that are used to reveal the relationships between the properties of biological or technical systems and multiple (measurable) control parameters. Multivariate methods are applied to classify observations and to identify the most discriminating parameters, thus reducing the dimensionality of a data set.
In the context of the biomaterial research at the IBP, I customize and apply multivariate methods, inclusive procedures for data preprocessing, to classify various types of biological samples. Emphasis of my current work is placed on the MVDA of ToF-SIMS spectra measured at decellularized extracellular matrices applying different variants of Principal Component Analysis (PCA), Discriminant Analysis of Principal Components (DAPC), Artificial Neuronal Networks (ANN), and Maximum Autocorrelation Factor (MAF).
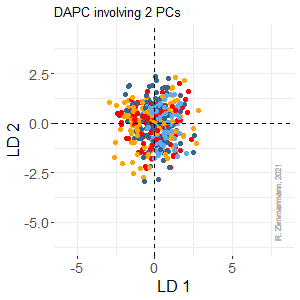